Research Data Repository of Saxon Universities
OPARA is the Open Access Repository and Archive for Research Data of Saxon Universities.
Researchers of Saxon Universities can either publish their research data on OPARA, or archive it here to comply with requirements of funding acencies and good scientic practice, without public access.
You can find the documentation of this service at the ZIH Data Compendium websites. If you need suppourt using OPARA please contact the Servicedesk of TU Dresden.
Please note: The OPARA service was recently upgraded to a new technical platform (this site). Previously stored data will not be available here immediately. It can be found at the still active old version of OPARA. These stock data will be migrated in near future and then the old version of OPARA will finally be shut down. Existing DOIs for data publications remain valid.
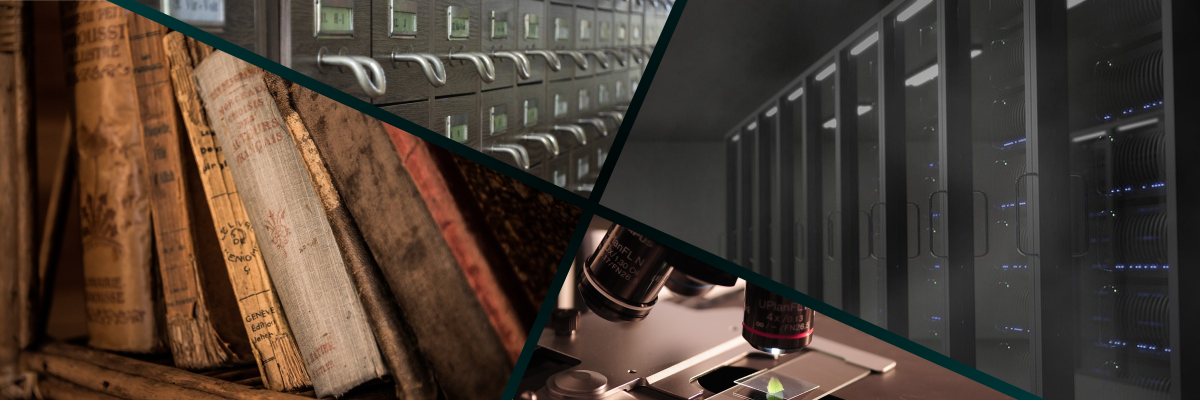
Communities in OPARA
Select a community to browse its collections.